Special thanks to Grant Quick for capturing Adriaan Steyn and me presenting this talk at Tableau Conference 2024.
The rise of artificial intelligence (AI) and big language models (LLMs) in data analysis has made me think about the future of data analysts. In this article, I'm going to talk about three ways AI can and is a threat to data analyst jobs, and suggest how we can adjust our careers to succeed in the world of AI.
Threat 1: AI and Analysts Do Similar Work
AI is increasingly able to do what data analysts do:
Preparing data
Analysing data
Visualising data
Even though the tools we use might be different, like Python, Tableau, Power BI, or Excel, the outcomes, providing insights, are increasingly similar. Whilst AI sometimes isn't as effective as human analysts today, it's improving fast and becoming smarter and more competent with each new release.
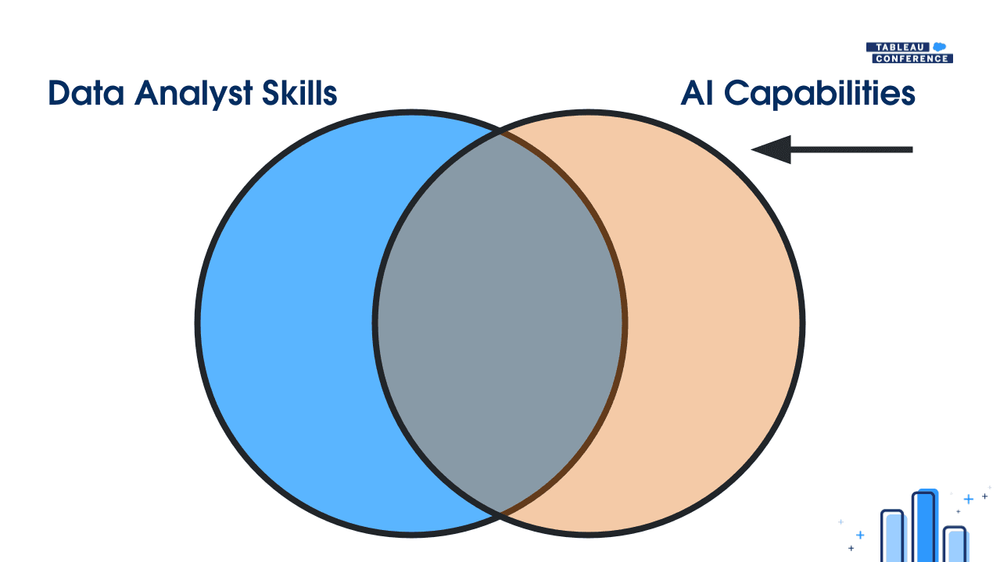
The overlap in what AI and analysts do is almost complete. If you look at a data analyst's job description, the only things left mainly for humans are communication and presentation skills - bad news for all the introverts out there! Given this, I can see the role of data analysts dying off and becoming an eventual function of AI.
Opportunity 1: The Data Analyst Upskilled with AI
Although AI and data analysts often do similar work, there's a big chance for analysts to grow their careers further. Just like calculators did away with the need for doing basic maths by hand, they didn't eliminate the need for maths skills -in fact, those skills are more sought after than ever. A calculator simply helps everyone do maths more easily and quickly.
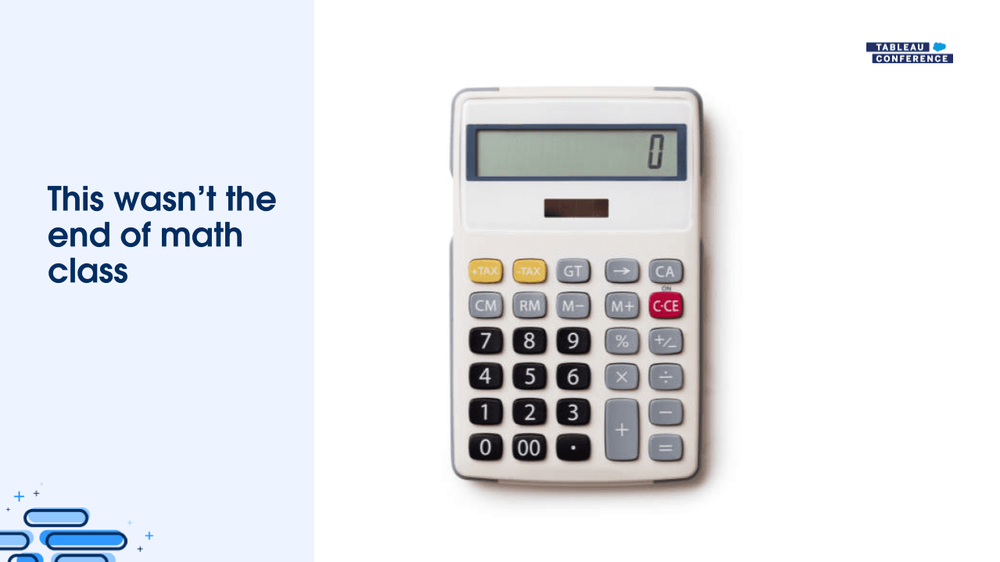
In the same way, a data analyst introducing a Tableau dashboard doesn’t mean the end of data analysis. Instead, it sets up a basic level of knowledge and understanding within the organisation, which actually leads to more questions and deeper analytics - data analysis becomes more in demand than ever before!
Could AI cut down the time it takes to complete routine tasks? We’ve seen instances in the community where AI aids analyst tasks, like Adam Mico’s custom GPT at OpenAI's GPT store, specifically designed to answer Tableau queries. This means you could avoid digging through Tableau's help pages and instead get quick, concise answers from a GPT.
If GPT-4o is available to you, you can now use tData Doctor GPT for free.
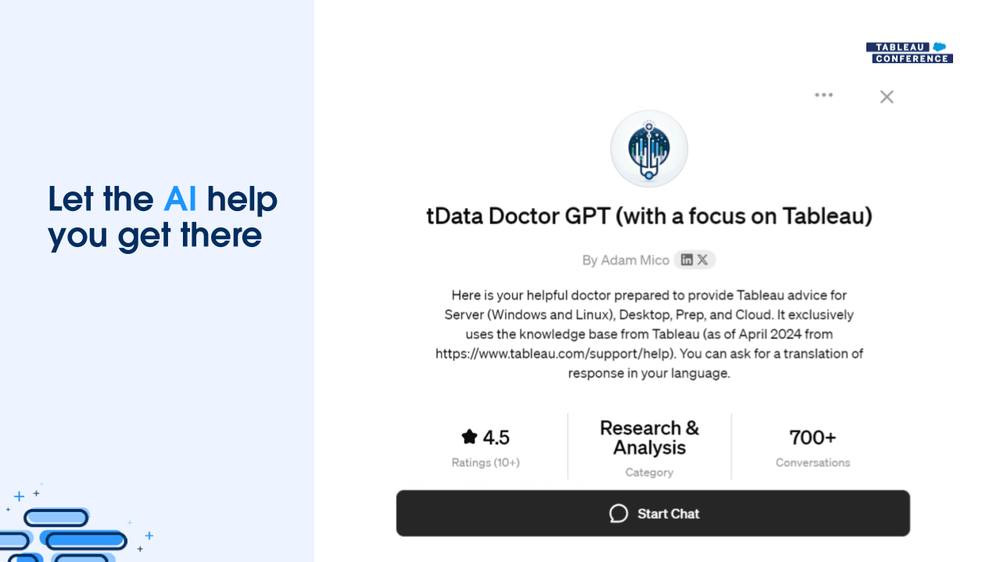
By mastering AI tools for their everyday tasks, analysts could save time to enhance their roles, such as:
Increasing domain knowledge: Moving closer to stakeholders and getting more involved in making decisions and forming business strategies.
Boosting technical skills: Exploring and developing more advanced technical solutions for the business.
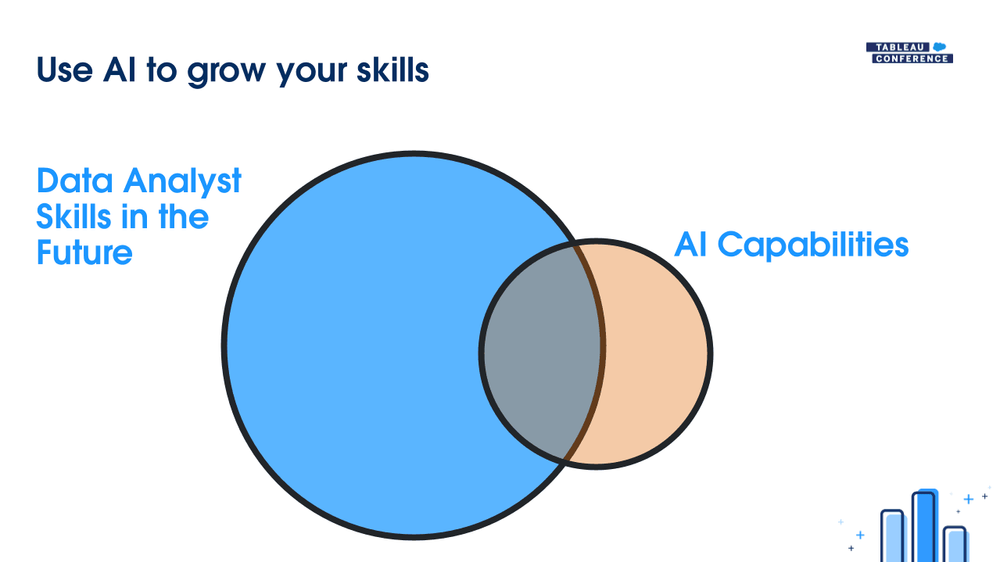
Threat 2: Stakeholders use AI and Analysts are out of the loop
Data is naturally messy and complicated to handle, making it hard to turn into clear answers or insights. As data analysts, our role is to help stakeholders understand this data, but it’s a time-consuming process. We usually create Tableau dashboards to help analyse the data and present our findings. In doing this we make data more accessible to many stakeholders and to address various questions. Throughout this process, analysts have control over the information being shared.
However, the rise of LLMs like ChatGPT has introduced a new way for stakeholders to access data. Companies are starting to use in-house LLMs or allow stakeholders to use publicly available AI models, as part of their routine work. This creates a risk for analysts: being disconnected from what stakeholders are asking.
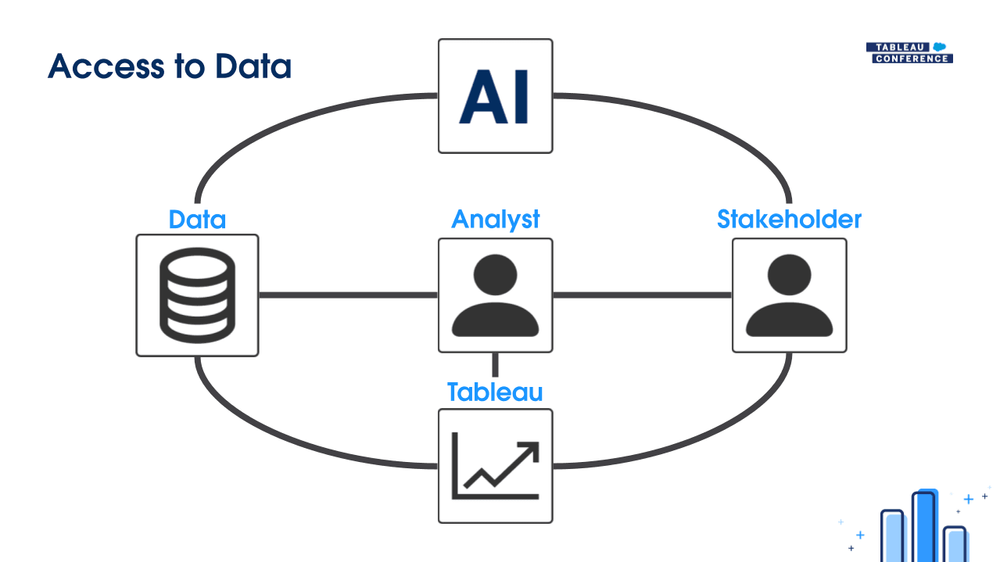
Because of this AI deployment, analysts don't know:
What questions stakeholders are asking
Whether the AI’s answers are accurate
If stakeholders find the AI responses useful
As companies push for AI to boost efficiency and performance, data analysts risk becoming disconnected from the stakeholders' needs. Making their roles 'out of touch' with the business and potentially, obsolete.
Opportunity 2: The Implementer of AI Solutions
Data analysts should be involved in how AI is used within their organisations. There are many parallels to deploying Tableau dashboards. When launching a dashboard, it's not just about setting up a solution.
Analysts need to:
Guide users how to interact with these tools,
Help them find and understand the data they need,
Gather feedback to enhance the tool for everyone.
These actions help build organisational trust in the tools and maximise their business value. The same will be true for deploying AI.
Tableau Pulse is an example of an AI solution that you could bring to stakeholders today. It greatly improves how quickly insights are delivered to stakeholders, but it still requires someone to develop, oversee, and maintain it to ensure it delivers real value.
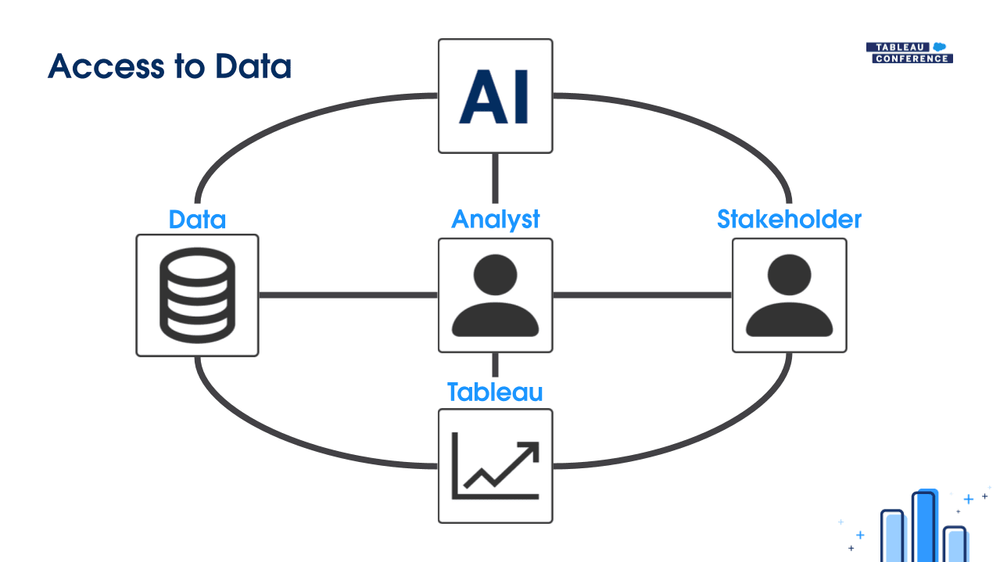
I believe analysts should expand their roles into that of AI solution deployers, managing and implementing these technologies, whether through dashboards or other interfaces. For instance, to assist a support team, an analyst could:
Create a dashboard to monitor ticket volumes over time, helping identify peak periods or delays, similar to Example dashboards
Implement an LLM model to generate initial responses to customer inquiries, just like GPT-4o from ChatGPT
Set up a contextual search module to speed up finding answers to frequent queries, like what Perplexity AI offers
These examples demonstrate practical ways analysts can integrate AI into their workflows, enhancing their strategic role and adding significant value to their organisations.
Threat 3: Too many tools and too many developments
While AI offers compelling advantages, the field is advancing incredibly fast. We now have AI models on our desktops, integrated into applications, ranging from paid to open-source versions, with new models continuously emerging. Just yesterday, OpenAI released GPT-4o, which is free and outperforms its predecessor, GPT-4.
Should we then shift all our workflows to this new model? Will the performance enhancement apply to our specific needs? What does this mean at an organisational level?
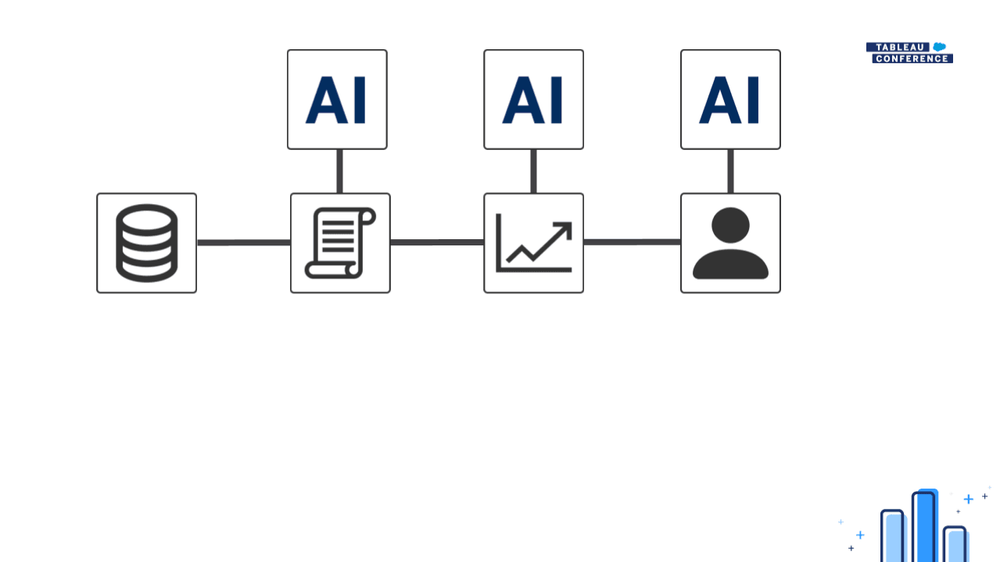
Consider a common scenario in an organisation where a stakeholder interacts with a dashboard. The process begins with:
Extracting data using a SQL script aided by GitHub Copilot,
Building the dashboard in Tableau with assistance from Einstein Copilot,
The stakeholder then uses ChatGPT to interpret the dashboard's results or to request further analysis from me.
This example of one stakeholder using one dashboard and three different AI tools illustrates the scalability challenges of implementing multiple AI technologies across an organisation.
Beyond the risk of tool overload, which can lead to inefficiencies and increased time spent testing AI systems - there are significant ethical concerns to consider. Ensuring that these AI technologies are 'fit for purpose' and ethically aligned is crucial. This means evaluating tools for transparency, fairness, data privacy, and the ability to mitigate biases.
Opportunity 3: The Need for the Tableau Community
We are not alone in the challenge of how to govern AI, select the right tools, and maintain control over AI deployments.
Think back to when you first started using Tableau. Although there was plenty of documentation and tutorials, much of your learning came from engaging with the community. Tableau Public has been a vital resource where you can find dashboards tailored to various business cases, download them, see how they were built, and learn from the community to improve your dashboard creations.
Why can't we replicate this model for AI solutions?
The Tableau community has proven the immense value of sharing knowledge and experiences. We could harness the same spirit to foster a robust AI governance community. This community could serve as a hub where AI practitioners share best practices, learnings, and even codebases to help each other navigate the rapidly evolving AI landscape.
Such a community could focus on several key areas:
Ethical AI Use: Establishing standards for ethical AI use within organisations, ensuring that AI solutions respect user privacy and are free from biases.
Effective AI Governance: Sharing strategies for effective oversight of AI tools, including performance monitoring and impact assessment.
AI Tool Integration: Offering guidance on integrating new AI tools with existing systems to enhance functionality without disrupting existing operations.
AI Education and Training: Providing ongoing education and training resources to help professionals stay up-to-date with new AI technologies and methodologies.
Conclusion
AI will significantly influence the roles of data analysts both now and in the future. To stay relevant and enhance their impact, analysts need to adapt by integrating AI into their workflows.
This includes upskilling with AI to streamline routine tasks, thereby freeing up time to expand and deepen their expertise. Analysts should also see themselves as implementers of AI solutions, ensuring these technologies deliver real value to their businesses, much like they do with dashboards. Moreover, by becoming community enablers who actively share their AI use cases and solutions, analysts can help shape standards for AI deployment and governance.
The AI + Tableau User Group
I am pleased to announce the AI + Tableau to help enable the collaboration of AI practices across the Tableau community. The user group is here to:
Ensure that we not only keep up with technology but also lead in its responsible implementation.
Develop a better understanding of what good AI governance looks like across different organisations
And help us implement AI solutions that are both innovative and trustworthy.
Click the link below to learn more about the user group